From automated pest and plant disease detection to intelligent spraying and produce sorting—here’s how Computer Vision is transforming the food and agriculture sector.
There’s no doubt that crop yields, quality, and labor practices are more efficient now than they were 500, or even 50 years ago.
However, there’s still a major need (and field) for improvements.
The global human population is exploding, with an estimated 9.9 billion of us on the planet by 2050 and with food demand projected to leap 35%—56% in that time. And that’s not to mention climatic changes that make resources like water and farmable land scarcer.
Luckily, technology provides us with yet another solution: AI.
From leveraging computer vision technology for crop and soil monitoring to disease detection and predictive analytics, the agriculture industry is entering a whole new phase of evolution—thanks to AI.
Not only is there potential, but also rapidly growing interest and investment:
- Forbes reports that global spending on “smart” agriculture, including AI and machine learning, is projected to triple to $15.3 billion by 2025.
- Research suggests that the market size of AI in agriculture should expect a compound annual growth rate (CAGR) of 20%, reaching $2.5 billion by 2026.
And that’s just the tip of the iceberg!
In this article, we’ll take a look at some of the most promising AI technologies transforming the agriculture sector.
Here’s what we’ll cover:
- Crop and soil monitoring
- Insect and plant disease detection
- Livestock health monitoring
- Intelligent spraying
- Automatic weeding
- Aerial survey and imaging
- Produce grading and sorting
- The future of AI in Agriculture: Farmers as AI engineers?
Crop and soil monitoring
Let’s start from the ground up.
Micro and macronutrients in the soil are critical factors for crop health and both the quantity and quality of yield.
Then, once crops are in the soil, monitoring the stages of growth is also essential to optimizing production efficiency. It’s vital to understand interactions between crop growth and the environment in order to make adjustments for improved crop health.
Now, traditionally soil quality and crop health were determined by human observation and judgment. But this method is neither accurate nor timely.
Instead, we can now use drones (UAVs) to capture aerial image data, and train computer vision models to use this for intelligent monitoring of crop and soil conditions.
Visual sensing AI can analyze and interpret this data to:
- track crop health
- make accurate yield predictions.
- detect crop malnutrition much faster than humans
AI models can inform farmers of specific problem areas so that they can take immediate action.
Now let’s look at some real examples of how computer vision is helping keep their crops healthy and productive.
Observing crop maturity
Manual observation of wheat head growth stages is just the kind of labor-intensive process that AI can help with in precision agriculture.
Researchers achieved this by collecting images of wheat at different “heading” stages across three years and in different lightings, which enabled them to create a “two-step coarse-to-fine wheat ear detection mechanism”.
This computer vision model was then able to outperform human observation in accurately identifying wheat growth stages, meaning that the farmers no longer had to make daily treks into the fields to examine their crop.
Or imagine having to check the ripeness of tomatoes by hand on an industrial level. Well..
AI can help with that, too!
Another study examined how well computer vision can detect maturity in tomatoes. Researchers created an algorithm that analyzed color from five different parts of the tomato, and then made maturity estimates based on this data.
How did it do?
Shockingly well! The algorithm achieved a successful detection and classification rate of 99.31%.
Overserving and estimating crop growth and maturity is hard, labor-intensive work for farmers. But AI is proving capable of handling much of that work with both ease and impressive accuracy.
Hitting the Ground with Computer Vision
Getting back to the importance of soil, another study set out to see how well computer vision can characterize soil texture and soil organic matter (SOM).
Ordinarily, evaluating soil requires farmers to dig up samples and bring them to a lab for time- and energy-intensive analysis. Instead, researchers decided to see if image data from an inexpensive handheld microscope could be used to train an algorithm to do the same thing.
Sure enough, the computer vision model managed to make sand content and SOM estimates with accuracy comparable to costly lab processing.
So, not only can computer vision eliminate a large amount of the difficult, manual labor involved in crop and soil monitoring, in many cases it does it more effectively than humans can.
Insect and plant disease detection
We’ve seen how AI computer vision can detect and analyze crop maturity and soil quality, but what about agricultural conditions that are less predictable?
Using image recognition technology based on deep learning, we can now automate detection of plant diseases and pests. This works using image classification, detection, and image segmentation methods to build models that can “keep an eye” on plant health.
Take a look at how this works:
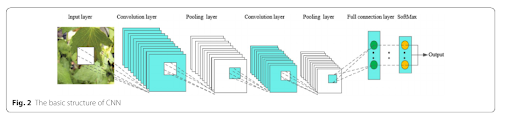
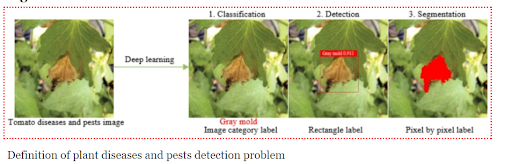
Keeping out the bad apples (diagnosing disease severity)
A good example of this in action comes from a study of apple black rot (which we’d all probably prefer off our apples!).
Researchers trained a Deep Convolutional Neural Network using images of apple black rot which had been annotated by botanists according to four major stages of severity.
As with our previous examples, the alternative to computer vision requires a lot of labor-intensive human searching and evaluation. Fortunately for farmers, the AI model in this study was able to identify and diagnose disease severity with an accuracy of 90.4%!
Researchers in another study went even further by using an improved YOLO v3 algorithm to detect multiple diseases and pests on tomato plants.
Armed with a digital camera and a smartphone, the researchers took photos at local tomato greenhouses and identified 12 different cases of either disease or pests.
Once the model was trained using the images, which varied in resolution and size of the object featured, it achieved a disease and pest detection accuracy of 92.39% with a detection time of just 20.39 ms.
Not too shabby!
Finding bugs with code
And say you’d like to know not only if your crops have pests, but how many there are, computer vision systems for insect detection has that covered as well.
Did we mention that this works for flying insects, too?
They’re certainly not the most fun to capture and count by hand.
Researchers first set up a sticky trap to capture six different species of flying insect and collect real-time images. They then based the detection and coarse counting method on YOLO object detection, and the classification and fine counting on Support Vector Machines (SVM) using global features.
When all was said and done, their computer vision model was able to identify bees, flies, mosquitoes, moths, chafers, and fruit flies with an accuracy of 90.18%, and count them with 92.5% accuracy.
These studies show that the future of AI computer vision for monitoring the health of our food systems is promising. Not only can it reduce labor inefficiencies, but it can do so without sacrificing reliability of the observations.
Livestock health monitoring
So far we’ve focused mainly on plants, but there’s more to agriculture than wheat, tomatoes, and apples.
Animals are another major component of our agriculture systems, and they tend to need a bit more tracking than plants. Can computer vision keep up with cows, chickens, and pigs on the move?
Well, if it can track a fly, it can certainly track a cow.
Check out below how one of V7’s users—CattleEye’s training data allows for tracking and annotating of cattle using bounding boxes and key points.
.png)
CattleEye is a great example of an AI-first company in the agriculture industry. They use overhead cameras and computer vision algorithms to monitor cattle health and behavior.
This means that spotting a problem isn’t dependent on a cattle farmer being right there next to the cow. Instead, the cattle can be tracked and monitored remotely and in real-time so that farmers can be notified as soon as a problem is observed.
Of course, this isn’t just limited to cattle. Computer vision can also:
- Count animals, detect disease, identify unusual behavior, and monitor significant activities such as giving birth.
- Collect data from cameras and drones (UAVs).
- Combine with other technologies to keep farmers informed on animal health and access to food or water.
Plus, take a look at how this image of chickens has been annotated with V7.
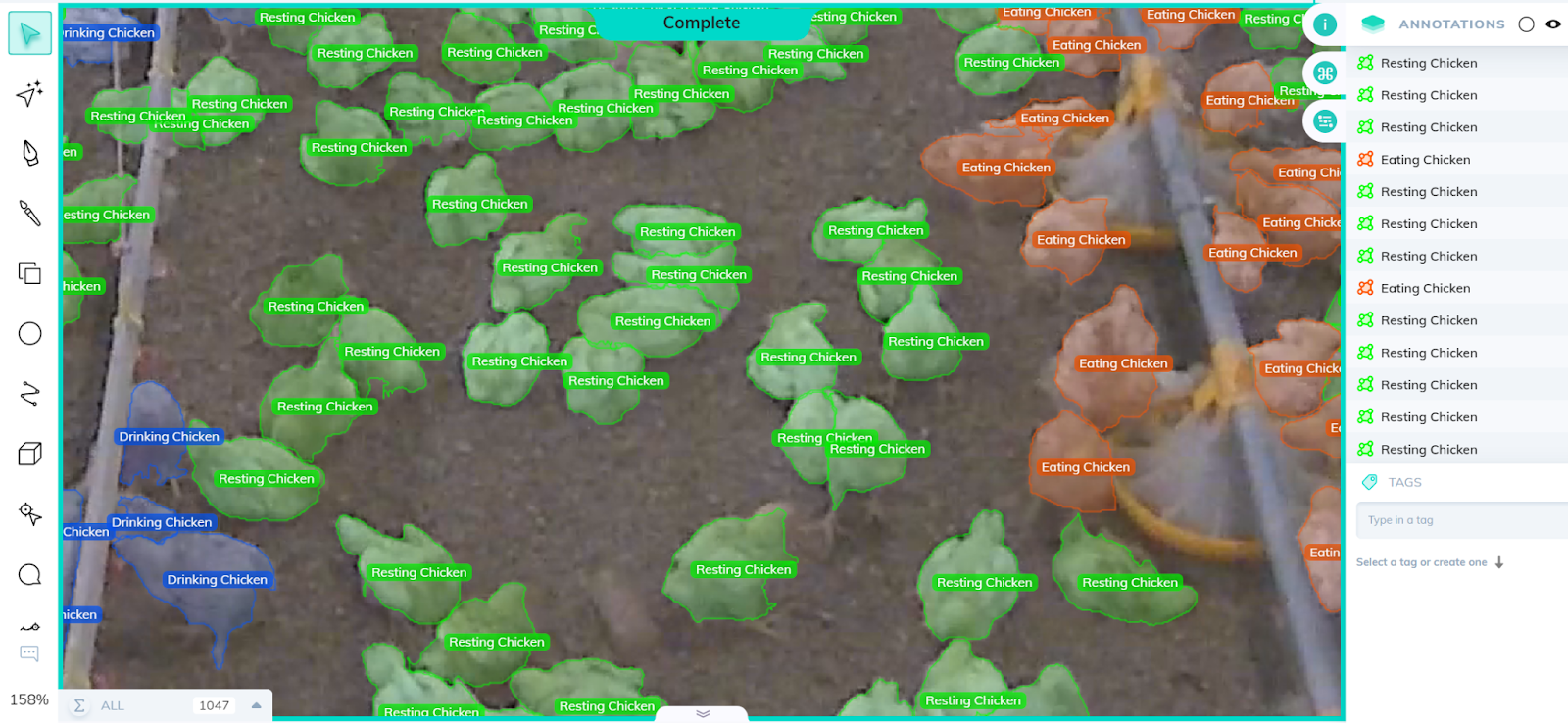
The algorithms are trained to look at video data and determine what the chickens are up to – whether they’re drinking, eating, sleeping, or doing something odd that may be indicative of disease or behavioral problems.
Intelligent spraying
We’ve seen that computer vision is good at spotting disorders in agriculture, but it can also help with preventing them.
UAVs equipped with computer vision AI make it possible to automate spraying of pesticides or fertilizer uniformly across a field.
With real-time recognition of target spraying areas, UAV sprayers are able to operate with high precision both in terms of the area and amount to be sprayed. This significantly reduces the risk of contaminating crops, humans, animals, and water resources.
While the potential here is great, currently some challenges still exist. For example, spraying a large field is much more efficient with multiple UAVs, but assigning specific task sequences and flight trajectories for individual crafts can be tricky.
But that doesn’t mean it’s game over for intelligent spraying.
Researchers from Virginia Tech have devised a smart spray system based on servo motor controlled sprayers that use computer vision to detect weeds. A camera mounted on the sprayer records the geo-location of weeds and analyzes the size, shape, and color of each pesky plant in order to deliver precise amounts of herbicide with precision targeting.
In other words, it’s a kind of weed terminator. But unlike the Terminator, the accuracy of the computer vision system allows it to spray with such accuracy that it manages to avoid collateral damage to crops or the environment.
Automatic weeding
Intelligent sprayers aren’t the only AI getting into weed… er, weeding. There are other computer vision robots taking an even more direct approach to eliminating unwanted plants.
Now, spotting a weed in the same way that computer vision can spot an insect or oddly-behaving chicken doesn’t actually eliminate very much work for the farmer. To be of even greater help the AI needs to both find and remove the weed.
Being able to physically remove weeds not only saves the farmer quite a bit of work, but also reduces the need for herbicides and thus makes the whole farming operation much more environmentally friendly and sustainable.
Robots in the weeds
Luckily, object detection can do a great job of identifying weeds and distinguishing them from the crops. However, the real power comes when computer vision algorithms are combined with machine learning to build robots that perform automatic weeding.
All this pretty well introduces BoniRob, an agricultural robot that uses camera and image recognition technology to find weeds and remove them by driving a bolt into the earth.
It learns to distinguish between weeds and crops through image training on leaf size, shape, and color. That way BoniRob can roll through a field eliminating undesirable plants without the risk of destroying anything of value.
And while our AI friends are out in the fields anyway, perhaps there are other jobs they could do.
A group of scientists is working on making this a reality with designs for agricultural robots that detect weeds as well as soil moisture content.
This way, it can move through a field, removing weed and delivering appropriate amounts of water to the soil as it goes.
Experimental results for this system show that its plant classification and weeding rates are both at or above 90%, all the while keeping deep soil moisture content at 80 ± 10%.
Ai-driven agriculture bots are developing quite the resume!
Aerial survey and imaging
At this point it’s probably unsurprising that computer vision also has some terrific applications for surveying land and keeping an eye on crops and livestock.
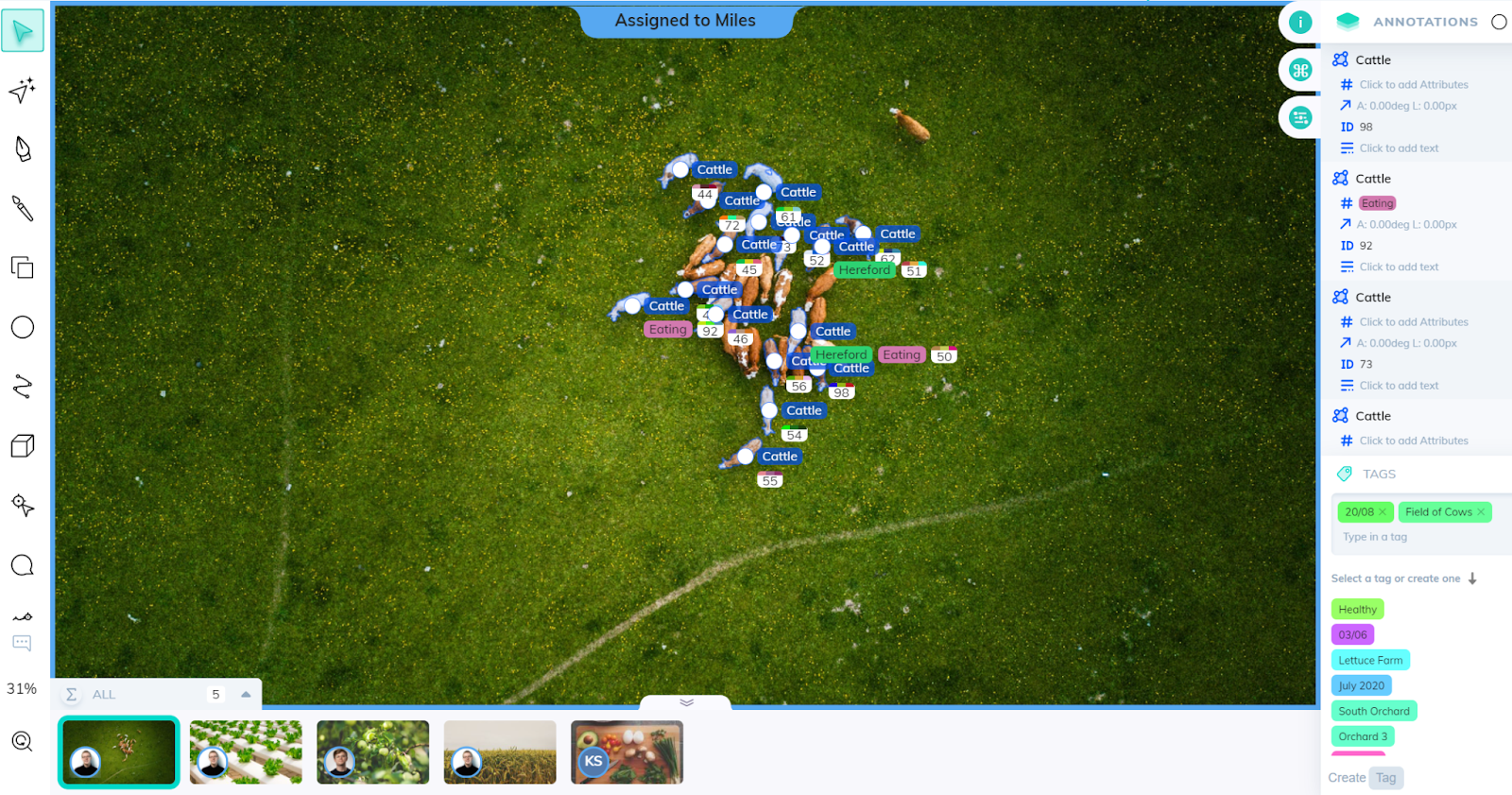
But that doesn’t make it any less significant for smart farming.
AI can analyze imagery from drones and satellites to help farmers monitor crops and herds. That way they can be notified immediately if something looks amiss without having to constantly observe the fields themselves.
Aerial imaging is also useful for boosting the precision and efficiency of pesticide spraying. As mentioned previously, ensuring that pesticides only go where they’re intended saves money as well as the surrounding environment.
Produce grading and sorting
Finally, AI computer vision can continue to help farmers even once the crops have been harvested.
Just as they are able to spot defects, disease, and pests as the plants are growing, imaging algorithms can also be used to sort “good” produce from the defective or just plain ugly.
By inspecting fruit and vegetables for size, shape, color, and volume, computer vision can automate the sorting and grading process with accuracy rates and speed much higher than even a trained professional.
Picture perfect produce
Take carrot sorting, for example.
It’s laborious and usually done by hand. However, researchers have developed an automated sorting system that uses computer vision to pick out carrots that have surface defects or are not the correct shape and length.
A “good” carrot, then, is one that’s the right shape (a “convex polygon”) and does not contain any fibrous roots or surface cracks.
On these three criteria, the computer vision model was able to sort and grade carrots with accuracy rates of 95.5%, 98% and 88.3%, respectively.
Further, bringing us back to the classic tomato, another study found that AI with machine learning was able to use image data with seven input features to grade tomato quality with 95.5% accuracy.
In both cases, the amount of painstaking manual labor saved is enormous. And it’s all thanks to a bit of AI training on what a “good” carrot or tomato looks like.
The future of AI in Agriculture: Farmers as AI engineers?
Throughout human history, technology has long been used in agriculture to improve efficiency and reduce the amount of intensive human labor involved in farming. From improved plows to irrigation, tractors to modern AI, it’s an evolution that humans and agriculture have undergone since the invention of farming.
The growing and increasingly affordable availability of computer vision stands to become another significant step forward here.
With considerable changes occurring in our climate, environment, and global food needs, AI has the ability to transform 21st century agriculture by:
- Increasing efficiency of time, labor, and resources.
- Improving environmental sustainability.
- Making resource allocation “smarter”.
- Providing real-time monitoring to promote greater health and quality of produce.
Of course, this will require some shifts in the agricultural industry. Farmers’ knowledge of their “field” will need to be translated into AI training, and this will depend on greater technical and educational investments within the agricultural sector.
But then again, innovation and adaptation are nothing new in agriculture. Computer vision and agricultural robotics are just the latest way farmers can adopt new technology to meet growing global food demands and increase food security.
source:https://www.v7labs.com/blog/ai-in-agriculture